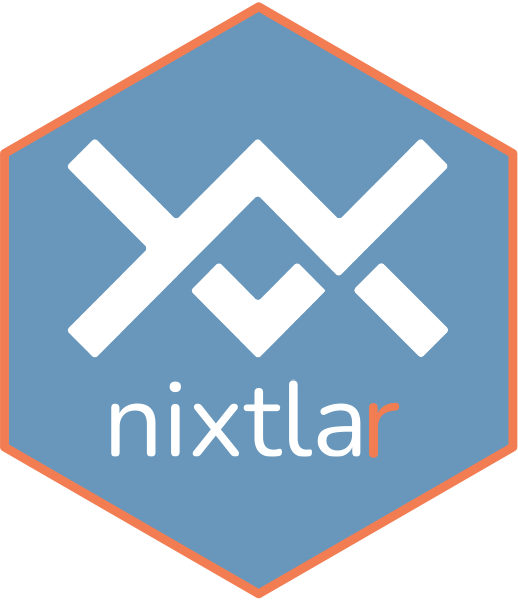
Sequential version of 'nixtla_client_cross_validation' This is a private function of 'nixtlar'
Source:R/nixtla_client_cross_validation.R
nixtla_client_cross_validation.Rd
Sequential version of 'nixtla_client_cross_validation' This is a private function of 'nixtlar'
Usage
nixtla_client_cross_validation(
df,
h = 8,
freq = NULL,
id_col = "unique_id",
time_col = "ds",
target_col = "y",
level = NULL,
quantiles = NULL,
n_windows = 1,
step_size = NULL,
finetune_steps = 0,
finetune_depth = 1,
finetune_loss = "default",
clean_ex_first = TRUE,
model = "timegpt-1"
)
Arguments
- df
A data frame with time series data.
- h
Forecast horizon.
- freq
Frequency of the data.
- id_col
Column that identifies each series.
- time_col
Column that identifies each timestep.
- target_col
Column that contains the target variable.
- level
The confidence levels (0-100) for the prediction intervals.
- quantiles
Quantiles to forecast. Should be between 0 and 1.
- n_windows
Number of windows to evaluate.
- step_size
Step size between each cross validation window. If NULL, it will equal the forecast horizon (h).
- finetune_steps
Number of steps used to fine-tune 'TimeGPT' in the new data.
- finetune_depth
The depth of the fine-tuning. Uses a scale from 1 to 5, where 1 means little fine-tuning and 5 means that the entire model is fine-tuned.
- finetune_loss
Loss function to use for fine-tuning. Options are: "default", "mae", "mse", "rmse", "mape", and "smape".
- clean_ex_first
Clean exogenous signal before making the forecasts using 'TimeGPT'.
- model
Model to use, either "timegpt-1" or "timegpt-1-long-horizon". Use "timegpt-1-long-horizon" if you want to forecast more than one seasonal period given the frequency of the data.
Examples
if (FALSE) { # \dontrun{
nixtlar::nixtla_set_api_key("YOUR_API_KEY")
df <- nixtlar::electricity
fcst <- nixtlar::nixtla_client_cross_validation(df, h = 8, id_col = "unique_id", n_windows = 5)
} # }